AI and cyclists work together for improved urban environments
Copenhagen is one of the world’s most bike-friendly cities, but ensuring safe and accessible transportation for cyclists can be costly and complicated. A Danish start-up have been testing a new solution at DOLL Living Lab that could make it both easier and more cost-efficient.
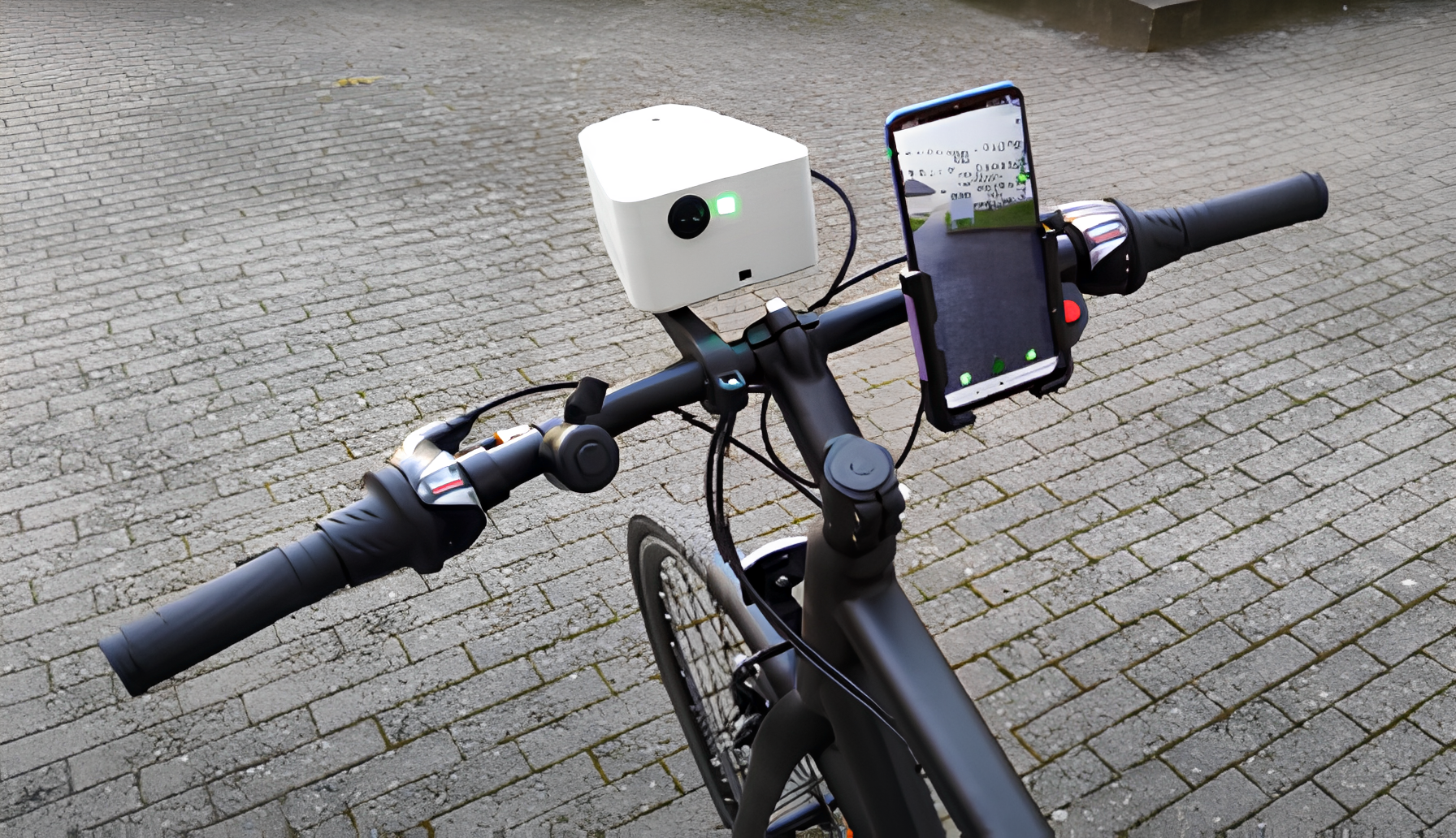
The AI-powered RoadSystem solution by Social Tech Projects
As is often the case with AI-based technology, it tends to work best in collaboration with humans. In this case, the network of cycle lanes around DOLL Living Lab gets scanned and monitored by sophisticated sensors mounted on a bicycle’s handlebar, with the cyclist manually covering the area by pedal.
Revolutionizing urban planning with deep learning
The system recognises different problems on the roads, from potholes and faded lane markings, to lighting conditions, noise and air pollution. This information gets sent to the datahub where the input provides training material for a deep-learning machine vision model. The correlated and validated data then gets analysed, with automated reports highlighting areas of special attention that can be sent to the relevant city department for effective planning, resource-allocation and policymaking.
The technology, developed by Social Tech Projects, has already demonstrated its adaptability across diverse urban environments. DOLL and CitCom.ai provide the space and services for also showcasing its capabilities in a smart city context. The model visualises the data in a user-friendly way through map-based insights and utilises cutting-edge algorithms for tasks like pothole detection and environmental monitoring. Their latest model, called ROAD System 365, provides a more holistic view of urban mobility challenges, including noise and air pollution monitoring.
“As a startup at the forefront of integrating AI with urban mobility, DOLL Living Lab’s testing environment and the CitCom.ai’s network puts us in a unique position to not only contribute to the safer roads initiative but also to contribute to the future of AI application in Europe,” says Luca Leomanni, CTO at Social Tech Projects.
The case highlights the potential effectiveness of data-driven approaches in urban planning. Key insights include the identification of areas for infrastructure improvement, the correlation between environmental factors and cyclist safety, and the potential for real-time data to inform policy and urban design decisions.
The power of partnerships in urban tech solutions
Social Tech Projects also raise the importance of collaborative efforts in solving complex urban challenges, the need for ongoing technological advancement, and the value of scalable solutions. Start-ups are often also dependent on networks and partnerships for scaling up solutions and staying up to date with evolving sensor technologies.
The ROAD System’s successful demonstration at the DOLL Living Lab illustrates the immense potential of testing and refining AI-driven solutions in real-world settings, paving the way for smarter, safer, and more sustainable cities.
“The machine-learning system requires a lot of layered data, but it’s the quality of the data that is key to its eventual success. We always operate on the basis that good data is local data.”
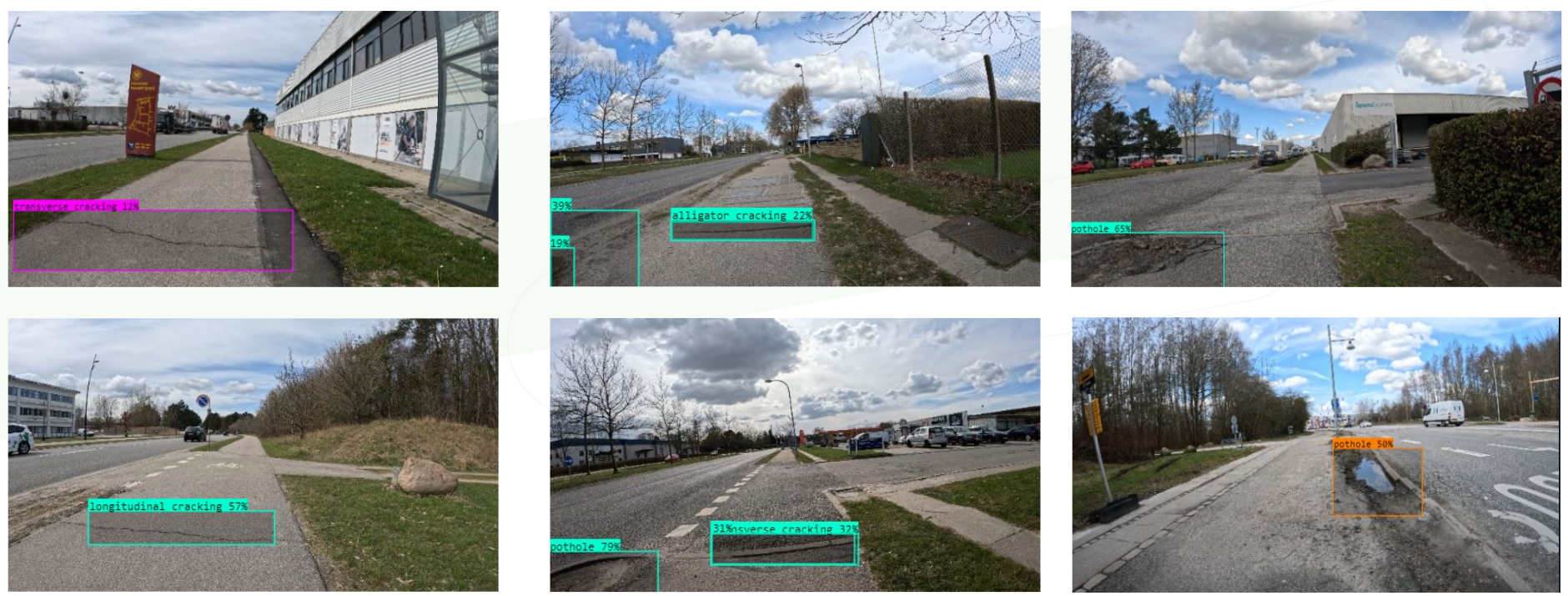
Examples of machine vision learning AI detection. Photo: Social Tech Projects
As is often the case with AI-based technology, it tends to work best in collaboration with humans. In this case, the network of cycle lanes around DOLL Living Lab gets scanned and monitored by sophisticated sensors mounted on a bicycle’s handlebar, with the cyclist manually covering the area by pedal.
Revolutionizing urban planning with deep learning
The system recognises different problems on the roads, from potholes and faded lane markings, to lighting conditions, noise and air pollution. This information gets sent to the datahub where the input provides training material for a deep-learning machine vision model. The correlated and validated data then gets analysed, with automated reports highlighting areas of special attention that can be sent to the relevant city department for effective planning, resource-allocation and policymaking.
The technology, developed by Social Tech Projects, has already demonstrated its adaptability across diverse urban environments. DOLL and CitCom.ai provide the space and services for also showcasing its capabilities in a smart city context. The model visualises the data in a user-friendly way through map-based insights and utilises cutting-edge algorithms for tasks like pothole detection and environmental monitoring. Their latest model, called ROAD System 365, provides a more holistic view of urban mobility challenges, including noise and air pollution monitoring.
“As a startup at the forefront of integrating AI with urban mobility, DOLL Living Lab’s testing environment and the CitCom.ai’s network puts us in a unique position to not only contribute to the safer roads initiative but also to contribute to the future of AI application in Europe,” says Luca Leomanni, CTO at Social Tech Projects.
The case highlights the potential effectiveness of data-driven approaches in urban planning. Key insights include the identification of areas for infrastructure improvement, the correlation between environmental factors and cyclist safety, and the potential for real-time data to inform policy and urban design decisions.
The power of partnerships in urban tech solutions
Social Tech Projects also raise the importance of collaborative efforts in solving complex urban challenges, the need for ongoing technological advancement, and the value of scalable solutions. Start-ups are often also dependent on networks and partnerships for scaling up solutions and staying up to date with evolving sensor technologies.
The ROAD System’s successful demonstration at the DOLL Living Lab illustrates the immense potential of testing and refining AI-driven solutions in real-world settings, paving the way for smarter, safer, and more sustainable cities.
“The machine-learning system requires a lot of layered data, but it’s the quality of the data that is key to its eventual success. We always operate on the basis that good data is local data.”
Looking ahead: predictive models for smarter cities
A year into its pilot phase, the project has already demonstrated its potential to transform urban planning. With trials conducted across diverse Danish cities, the system has been validated for its adaptability, proving valuable in testing the model’s reproducibility in other urban environments. The next phase aims to introduce a predictive maintenance model capable of forecasting future road conditions, enabling cities to allocate resources more efficiently.
Testing the solution at DOLL Living Lab has also enabled Social Tech Projects to focus on the system’s interoperability for data exchange, through Fiware’s standard protocol. The collaboration with KMD, another DOLL-partner, the solution can also be integrated into the IoT-platform of Danish municipalities.
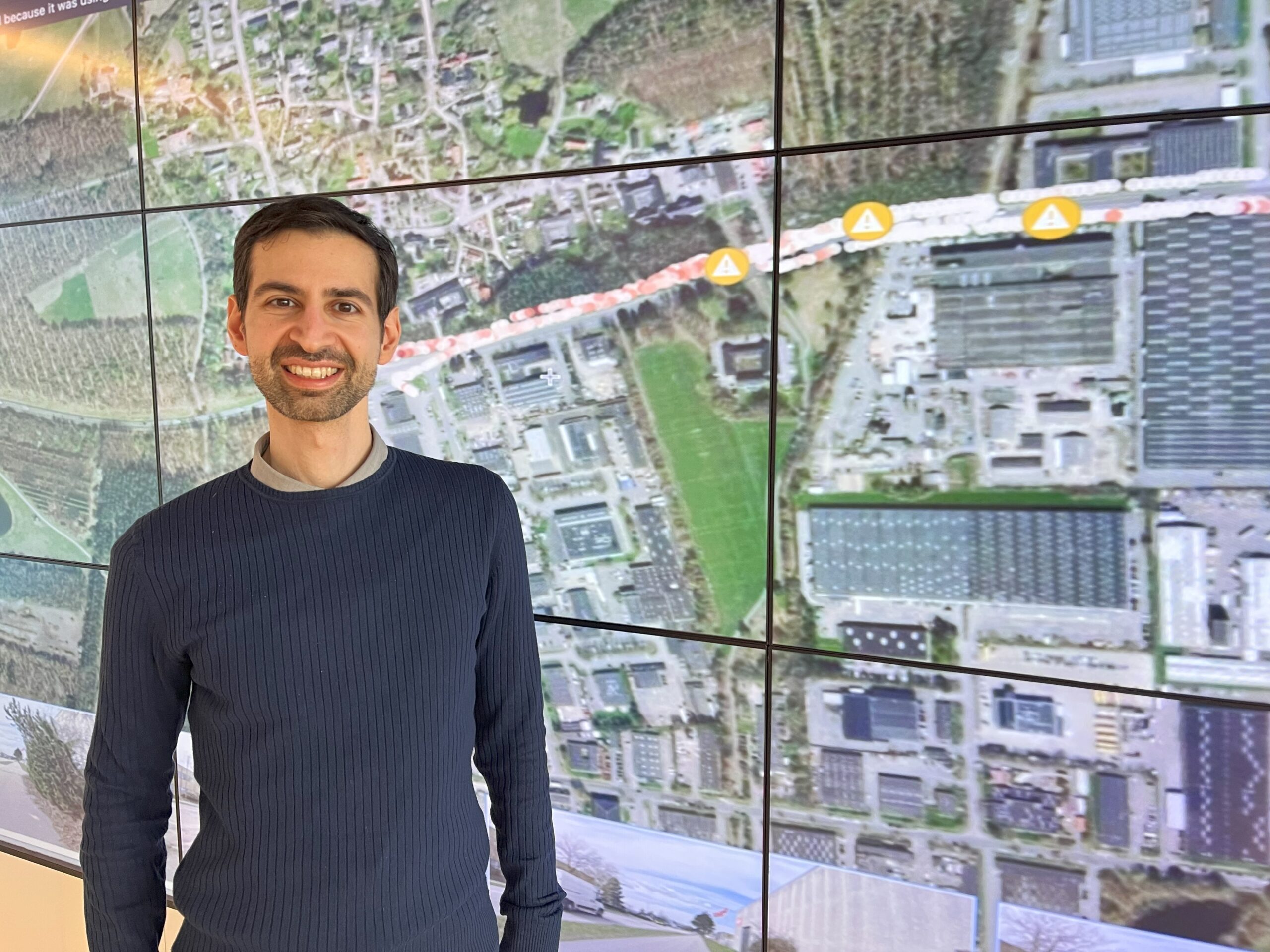
Luca Leomanni, CTO at Social Tech Projects
We invite AI innovators, public sector entities, and technology enthusiasts to join us in this new era of Smart City development. For more information, please contact Project Manager, Clara Hvidt Røder, clara.roeder@webuilddenmark.dk.
FACTS ABOUT CITCOM.AI
CitCom.ai is one of four so called TEFs – specialised large-scale reference sites to test state-of-the-art AI and robotics solutions. They are open to all European technology providers wanting to test both soft-and hardware AI products and services in.
CitCom.ai is the TEF for smart cities and communities.
- Led by DTU
- Budget: €40.000.000
- Consortium: 33 European partners offering sites to test AI
- Project duration: 2023-2027 (60 months)
Clara Hvidt Røder
Project manager clara.roeder@webuilddenmark.dk +45 25 53 55 43
Recent Comments